Unlocking Business Growth Through Machine Learning Data Annotation
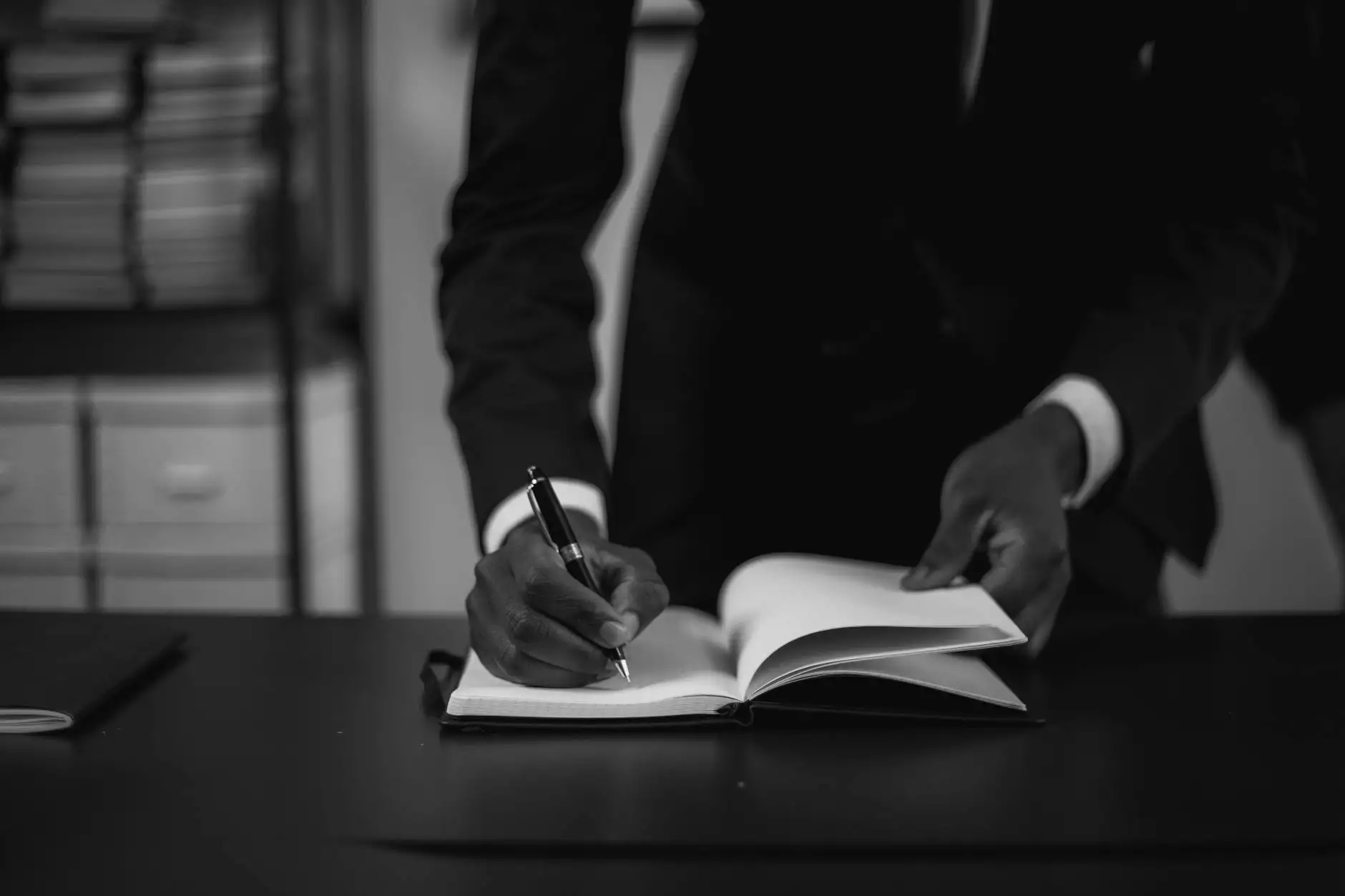
In today's fast-paced digital landscape, businesses are constantly seeking innovative solutions to enhance their service delivery and operational efficiency. One such breakthrough technology is machine learning data annotation, which has the potential to transform various industries, including Home Services, Keys & Locksmiths, and many others. This article delves into the nuances of data annotation, its significance in the machine learning ecosystem, and how it can drive growth in business.
What is Machine Learning Data Annotation?
Machine learning data annotation is the process of labeling data to help machine learning models learn from it. This input is crucial for creating accurate models, which can subsequently perform tasks such as image recognition, text analysis, and more. Without properly annotated data, machine learning algorithms struggle to understand patterns and make predictions.
The Role of Data Annotation in Machine Learning
Data annotation serves as the foundation for machine learning models. The more accurately data is labeled, the better the model can interpret and generalize from that data. Common types of data annotation include:
- Image Annotation: Identifying objects, actions, or facial expressions within images.
- Text Annotation: Tagging parts of speech, entities, sentiments, and more in text.
- Audio Annotation: Labeling sounds, emotions, or speech in audio clips.
- Video Annotation: Tagging objects and activities in video data.
Importance of Data Annotation for Businesses
The application of machine learning data annotation is not limited to tech firms. Businesses across various domains are leveraging this technology to enhance their operations:
1. Improved Decision Making
With accurate data labeling, businesses can train models that analyze trends and customer behavior, leading to informed strategic decisions.
2. Enhanced Customer Experience
Customizing services based on consumer data can significantly improve customer satisfaction. For example, locksmiths can leverage data to understand common service requests, optimizing their offerings to meet customer needs.
3. Increased Efficiency
By automating data-driven tasks with machine learning, businesses can improve operational efficiency and reduce human error, leading to faster service and cost savings.
The Data Annotation Process
The journey of machine learning data annotation begins with raw data. This unstructured data typically lacks context and structure and needs to be prepped for machine learning algorithms. The process involves several critical stages:
Step 1: Data Collection
Businesses must gather a diverse dataset relevant to their specific needs. This can include images, text, audio, or video data.
Step 2: Choosing the Right Annotation Tool
The efficiency of the annotation process heavily relies on the right tools. Various software solutions are available that streamline the annotation process, providing interfaces designed for speed and accuracy.
Step 3: Annotation Task Assignment
Data can be annotated by human annotators, automated tools, or a combination of both. For complex tasks, expert annotators may be required to ensure high accuracy.
Step 4: Quality Assurance
After the annotation, rigorous quality checks must be conducted to verify the accuracy of the labeled data. This step is essential for maintaining the integrity of the machine learning models.
Step 5: Retraining Models
With new and properly annotated data, businesses can continually retrain their models, enhancing their capabilities over time.
Challenges in Machine Learning Data Annotation
Like any technology, machine learning data annotation comes with its own set of challenges:
- High Labor Costs: The process can be time-consuming and labor-intensive, requiring skilled annotators.
- Quality Control: Ensuring consistent quality in annotations can be difficult, especially with larger datasets.
- Data Privacy: Annotators must handle sensitive information responsibly to comply with privacy regulations.
Leveraging Data Annotation for Home Services and Locksmiths
In the Home Services and Keys & Locksmiths sectors, machine learning data annotation can be particularly beneficial. Here is how:
1. Optimizing Service Delivery
Locksmiths can use annotated data to train models that help predict peak service times or the most common types of services requested. This allows businesses to allocate resources more effectively.
2. Enhancing Marketing Strategies
By analyzing customer data through machine learning models, locksmiths can fine-tune their marketing efforts, targeting specific audiences with personalized messages and services.
3. Automated Customer Support
Chatbots powered by machine learning can be trained to understand customer queries about services, pricing, and availability, providing immediate responses and improving customer satisfaction.
The Future of Machine Learning Data Annotation
The future of machine learning data annotation is promising. Emerging technologies and methods are set to streamline the process:
- AI-Based Annotation Tools: Innovations in AI will enable semi-automated data annotation, reducing the human effort required.
- Collaborative Annotation: Platforms allowing teams to work together on data annotation will enhance accuracy and efficiency.
- Continuous Learning: Machine learning models will increasingly adopt continuous learning, where they can adapt and improve based on real-time feedback and new data.
Conclusion
As businesses navigate the complexities of the modern market, embracing innovations such as machine learning data annotation can set them apart from competitors. By leveraging this technology, companies in the Home Services and Keys & Locksmiths sectors can improve decision-making, enhance customer experiences, and drive operational efficiency.
The journey to harnessing the full potential of machine learning starts with reliable and accurately annotated data. Businesses that invest in this process today will undoubtedly reap the rewards in the future, paving the way for sustained growth and success in an increasingly data-driven world.