Unlocking Potential: The Role of Annotation in Machine Learning
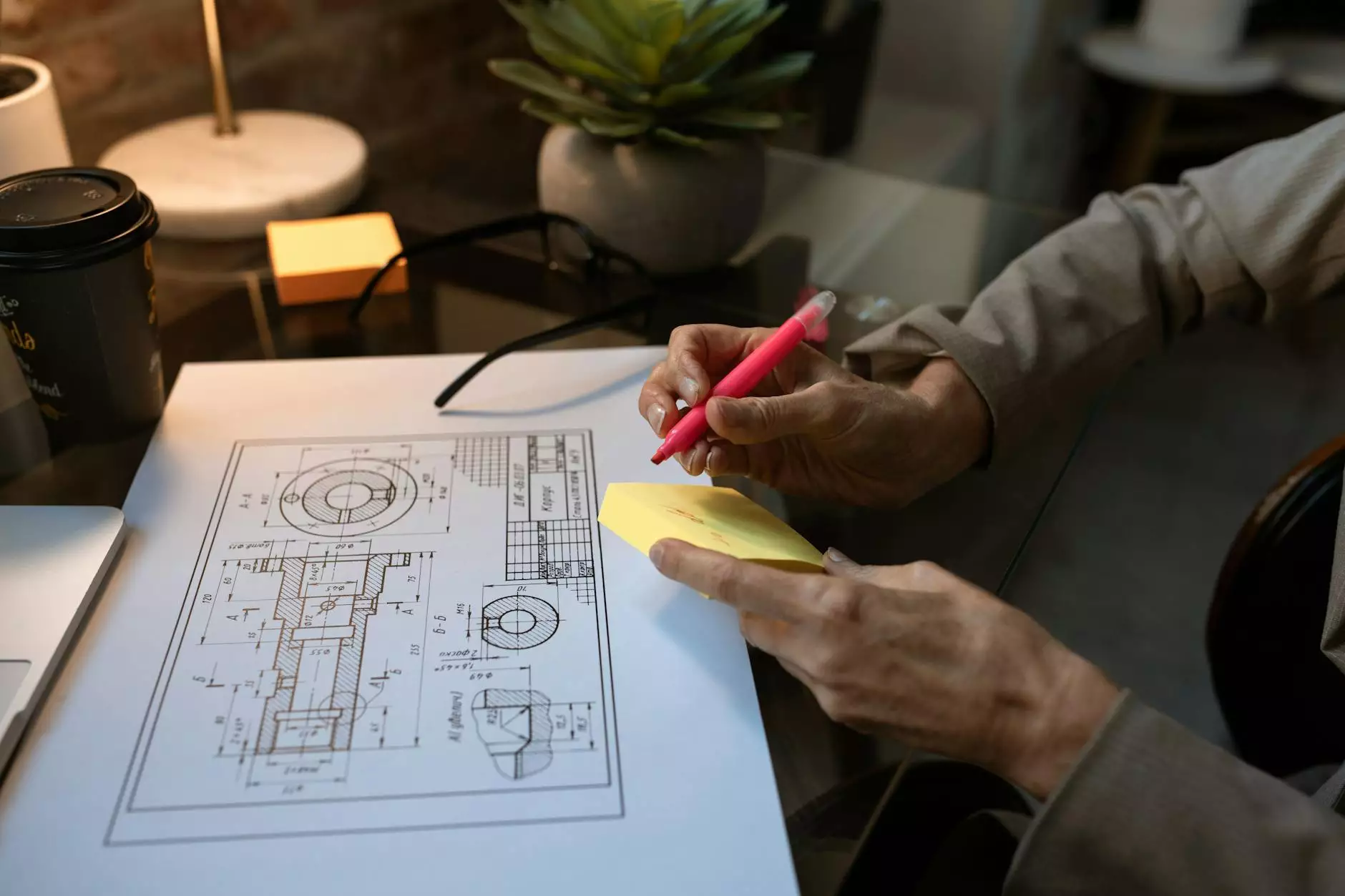
Machine learning has revolutionized countless industries, from health care to finance, making it a pivotal element in modern business operations. One of the most critical components of successful machine learning projects is annotation. The practice of data annotation, particularly in the context of machine learning, involves labeling datasets to train algorithms effectively. In this article, we will explore how annotation in machine learning can significantly enhance the efficiency and accuracy of business operations within fields such as Home Services, Keys & Locksmiths.
Understanding Machine Learning and the Importance of Annotation
Before delving into the specifics of how annotation affects business sectors, it’s essential to grasp the fundamentals of machine learning. At its core, machine learning is a branch of artificial intelligence that focuses on building systems that learn from data. By analyzing vast amounts of information, these systems can identify patterns, make decisions, and improve their performance over time.
The Lifeblood of Machine Learning: Data Annotation
Data annotation is the process of labeling or tagging data so that machine learning models can understand it. The necessity for high-quality annotated data cannot be overstated, as:
- Accuracy: Annotated data allows machine learning models to learn with high precision.
- Quality Training: Models trained on well-annotated datasets perform better compared to those trained on raw, unannotated data.
- Real-World Applications: The effectiveness of a model in real-world applications hinges on quality annotation.
How Annotation Machine Learning Benefits Home Services and Locksmith Industries
The Home Services and Keys & Locksmiths industries can significantly benefit from machine learning applications fueled by robust annotation processes. Below, we outline specific ways these industries leverage annotation for improved service delivery.
1. Enhanced Customer Service Through Predictive Analytics
In the realms of home services and locksmithing, understanding customer needs is paramount. By utilizing annotation machine learning, businesses can develop predictive analytics systems that analyze customer data patterns, thereby improving service responses.
- Customer Interaction Analysis: Annotated data from customer interactions can be used to predict future inquiries and allocate resources efficiently.
- Service Customization: By analyzing past customer choices and feedback, businesses can tailor services to meet specific preferences.
2. Optimizing Inventory and Supply Chain Management
Businesses in the Home Services and locksmith sectors often deal with various supplies and tools that need to be managed effectively. With machine learning models trained on well-annotated inventory data, organizations can:
- Forecast Demand: Predict which tools and keys will be in demand at given times, allowing for timely procurement and avoidance of overstock.
- Minimize Waste: Understand usage patterns leading to more efficient resource allocation, thus minimizing waste.
3. Security Insights through Image and Video Analysis
The use of surveillance systems in the locksmith industry can be enhanced through machine learning capabilities. By annotating video feeds and images, businesses can:
- Identify Security Threats: Machine learning models can be trained to recognize suspicious behaviors and alert security personnel.
- Analyze Access Patterns: Understanding how often and when customers access services can help improve security measures.
The Process of Data Annotation in Machine Learning
Implementing effective data annotation in machine learning involves several stages. Here’s a breakdown of the typical data annotation process:
- Data Collection: Gather a substantial dataset relevant to the specific use case.
- Defining Annotation Guidelines: Establish clear rules and standards for how data should be annotated.
- Annotation Execution: Utilize either human annotators or automated tools to label the data according to the established guidelines.
- Quality Assurance: Regularly review annotated data to ensure consistency and accuracy.
- Data Preprocessing: Clean and prepare the data for machine learning model ingestion.
Challenges in Data Annotation: Overcoming Potential Hurdles
While the benefits of annotation in machine learning are substantial, challenges do exist. Addressing these challenges is vital for maximizing the effectiveness of machine learning applications.
1. High Costs and Time Consumption
Annotating large datasets can be resource-intensive, both in terms of time and finances. Businesses must allocate adequate resources to ensure comprehensive data coverage. However, utilizing automation tools can help mitigate these costs.
2. Quality of Annotations
Ensuring high-quality annotations is essential. Mislabeling can lead to biased models and inaccurate predictions. Businesses should invest in continuous training for annotators and implement rigorous quality checks.
3. Keeping Up with Changing Data
The dynamic nature of data means that machine learning models and their associated annotations must evolve over time. Regular updates to datasets and annotations are crucial to maintaining model accuracy and relevance.
The Future of Annotation in Machine Learning
Looking ahead, the future of annotation machine learning appears promising, particularly in the Home Services and Keys & Locksmiths sectors. Emerging trends include:
- Automated Tools: Advancements in machine learning are leading to more sophisticated automated annotation tools that reduce the need for extensive human input.
- Integration with AI: Enhanced integration between AI technologies and data annotation processes is likely to streamline workflows and improve data quality.
- Increased Demand for Custom Solutions: As businesses seek tailored solutions, the need for specialized data annotation services is expected to grow.
Conclusion
In conclusion, the intersection of annotation machine learning and business practices within the Home Services and Keys & Locksmiths industries is a realm ripe with opportunity. High-quality annotated data empowers businesses to leverage machine learning technologies effectively, enhancing customer service, optimizing inventory, and increasing overall operational efficiency.
As we move further into the age of data-driven decision-making, investing in robust data annotation practices will undoubtedly yield competitive advantages, unlocking the full potential of machine learning solutions designed to meet evolving market demands.