The Importance of Image Annotation Tools in Machine Learning
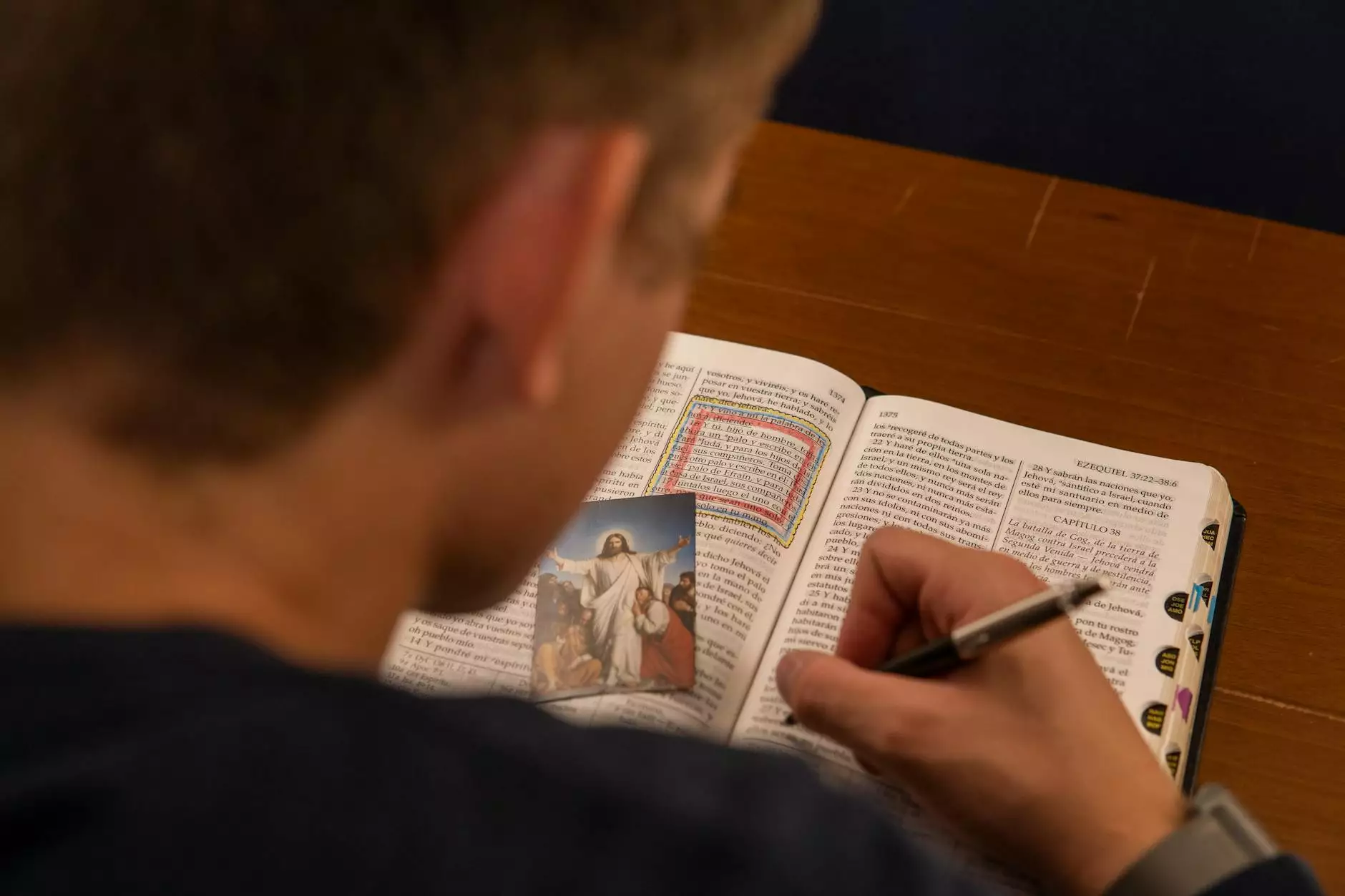
In the rapidly evolving world of technology, machine learning is becoming a cornerstone for innovation across various industries. One critical aspect that fuels the effectiveness of machine learning algorithms is the quality of the training data they utilize. This is where the role of image annotation tools comes into play. These tools are not just accessories in the machine learning toolkit; they are pivotal for developing accurate and efficient machine learning models.
What is Image Annotation?
Image annotation is the process of labeling images with relevant information, enabling machine learning models to interpret and understand the data effectively. This process involves marking objects, identifying features, and providing context to help algorithms learn from visual data. The annotated data serves as the foundation for training models in tasks such as object detection, image classification, and segmentation.
The Role of Image Annotation Tools in Machine Learning
Machine learning thrives on high-quality, accurately labeled data. Image annotation tools are instrumental in providing this crucial data. Here’s how:
1. Enhancing Data Quality
High-quality annotations ensure that machine learning algorithms can learn from reliable and relevant data. This directly impacts the performance of models, leading to improved accuracy and efficiency. With KeyLabs.ai’s advanced annotation techniques, users can expect:
- Accuracy: Precise labeling reduces errors in training data.
- Consistency: Uniform annotation ensures the model learns effectively across varied datasets.
- Scalability: Tools enable annotation of large datasets timely and efficiently.
2. Supporting Multiple Annotation Types
There is no one-size-fits-all approach when it comes to image annotation. Different machine learning applications require various annotation styles, such as:
- Bounding Boxes: Encloses objects within boxes to teach algorithms about their positioning.
- Semantic Segmentation: Labels each pixel of an image, useful in tasks requiring fine-grained image understanding.
- Keypoint Annotation: Marks specific features or joint locations on objects, crucial for use cases in medical imaging and engineering.
Types of Image Annotation Tools
When considering implementing an image annotation tool for machine learning, it's important to understand the various types available:
1. Manual Annotation Tools
These tools require human effort to label images. Although time-consuming, manual annotation often results in the highest accuracy. Manual tools can be beneficial for:
- Small datasets where high precision is necessary.
- Iterative processes where feedback is essential.
2. Automated Annotation Tools
With advancements in artificial intelligence, automated tools can streamline the annotation process. They leverage pre-trained models to suggest annotations. These tools are helpful for:
- Large datasets where efficiency is critical.
- Initial labeling that can later be refined manually.
3. Collaborative Annotation Platforms
These platforms allow multiple users to contribute to the annotation process, enhancing productivity. Such platforms often come with features for:
- Real-time collaboration.
- Quality control mechanisms to maintain data integrity.
Advantages of Using KeyLabs.ai for Image Annotation
KeyLabs.ai stands out as a premier provider of data annotation tools. Here’s why it is a top choice for enterprises looking to enhance their machine learning capabilities:
Precision and Accuracy
With a focus on delivering exceptional quality, KeyLabs.ai employs experienced annotators who ensure that every image is labeled with the utmost precision. This attention to detail is vital for creating effective machine learning models.
Customizable Solutions
Every business is unique, and KeyLabs.ai acknowledges this by offering customizable annotation solutions tailored to specific project requirements. This flexibility allows clients to dictate the annotation style, tools used, and more, resulting in a product that meets their needs perfectly.
Fast and Efficient Turnaround
Time is of the essence in machine learning projects. KeyLabs.ai utilizes cutting-edge technology to ensure rapid turnaround times without compromising on quality. This capability keeps projects on schedule and within budget.
Best Practices for Image Annotation
To maximize the efficacy of image annotation for machine learning, consider the following best practices:
1. Define Clear Annotation Guidelines
Establish detailed guidelines for annotators to ensure consistency and accuracy. Clear instructions minimize ambiguity and lead to better quality data.
2. Use a Diverse Dataset
Diverse datasets help models generalize better across different scenarios. Include a wide variety of images to cover all possible scenarios the model may encounter.
3. Iterative Annotation Review
Regularly review annotated data to identify any discrepancies or areas for improvement. Implementing feedback loops can significantly enhance data quality over time.
Conclusion: The Future of Image Annotation in Machine Learning
The future of image annotation tools in machine learning is bright, especially with the rise of advanced technologies and methodologies. As the demand for high-quality data continues to surge, businesses that harness the power of these tools will have a distinct competitive advantage.
At KeyLabs.ai, our commitment to providing cutting-edge data annotation platforms ensures that organizations are equipped with the necessary tools to succeed in machine learning endeavors. Embrace the power of image annotation tools and unlock the full potential of your machine learning projects today!
Get Started Today!
Interested in transforming your machine learning projects with superior image annotation capabilities? Visit KeyLabs.ai and let us help you enhance your data for the best results in your AI initiatives.
image annotation tool machine learning